

For example, one goal of the scheduling process is to get the best match-ups in the TV time slots that have the widest possible audience. This shift is important, since building a great schedule can be as much art as science. Before, the question facing the planning team was simply, “Can we just get it done?” Now, it is about finding the best possible schedule. The focus migrated from schedule creation (which by itself is far more complicated than it was only a few years ago) toward schedule analysis. With the switch to computers and the increasing power of optimization, a dramatic shift happened. The remainder was spent tweaking that feasible schedule to improve either a specific team or network’s schedule. The original goal was simply to find a feasible schedule and accomplishing that often consumed up to 90 percent of the available planning time. Hung on a wall the board consisted of a grid with 17 rows representing each week of the season and 32 columns representing each team. Until the last decade, a wooden six-foot square board was used to map out who would play whom, when and where. Making the problem particularly hard is the addition of numerous “additional” constraints including not scheduling teams from shared markets, such as the NY Jets and Giants, to play on the same day/time, ensuring no team has more than three road games in a row, and increasing the importance of late-season games by scheduling as many divisional match-ups as possible in the final weeks. A data scientist would take years to determine a good schedule out of that huge number of possibilities. Imagine the number one followed by 19 zeros. However, when you work it out, the number of possible schedules is well into the trillions. To some, that may seem like a lot of planning time available for seemingly few decisions.
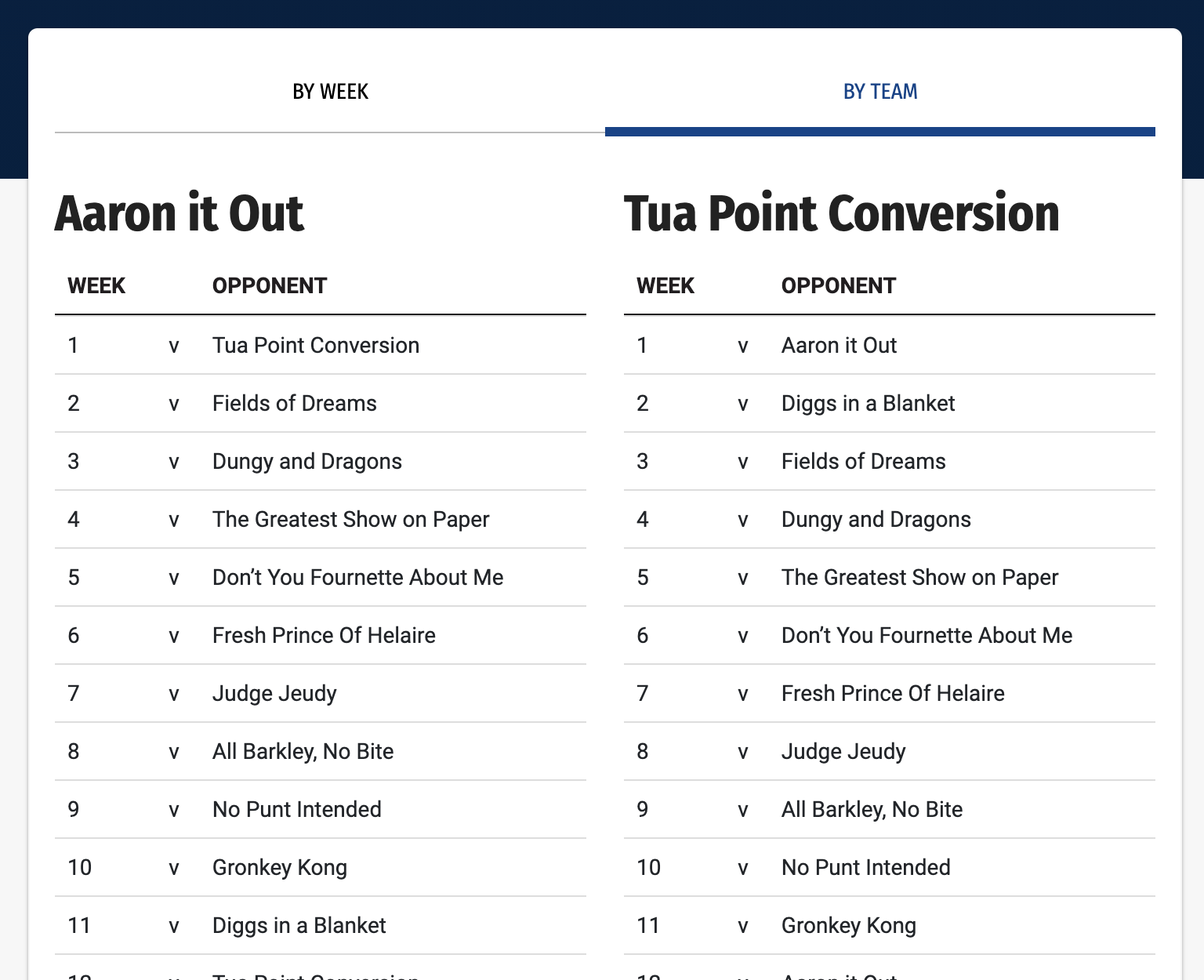
Four people have 10 weeks to schedule 256 games over the course of a 17-week season. The US’s National Football League is a perfect example of this. Of course, the response to this advancement has been to tackle ever larger and harder problems. Over the past few decades optimization has made huge strides what were once unsolvable problems are now solved in only seconds.
